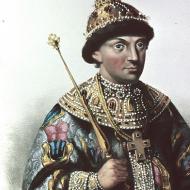
Shelf display: merchandising problems. “Black” and “white” merchandising: the war for shelf space What shelf space is needed for
When planning the retail space of a store, its owner faces a number of questions. How to make product display varied, but not redundant? How to ensure that the display attracts as many customers as possible, and poorly selling products do not take up space on the shelf? How to calculate the optimal stock on the shelf so as not to “freeze” extra money, but at the same time so that the product the buyer needs is always present in the store and in sight? Tools for optimizing shelf space successfully solve these problems.
Creating planograms using GOLD Space Planning and GOLD Space Automation
Optimizing shelf space on racks improves product availability and helps reduce the number of low-turnover products on the shelf. The assortment is displayed on the shelf in accordance with the company’s uniform merchandising rules. During optimization, factors such as the specifics of store equipment, unique sales data, and local store features are taken into account.
Tools are used to optimize shelf space GOLD Space Planning and GOLD Space Automation.
GOLD Space Planning– a tool for creating planograms and analyzing their effectiveness.
GOLD Space Automation– a tool for automatically generating unique planograms for each store based on templates or rules.
By using GOLD Space Planning you will be able to visualize retail equipment and product placement in 2D and 3D. The program allows you to determine the required level of inventory for assessment and optimization of display.
The user is provided with ample opportunities for preparing merchandising reports and managing retail equipment.
Creating planograms is as simple as possible: you simply drag and drop products from the database onto the shelves.
As in GOLD Macro Space Planning, “hot” and problem areas in Space Planning are highlighted. This helps optimize the placement of product items.
GOLD Space Planning has advanced functionality for maintaining a variety of merchandising reporting. The program provides a function for comparing planograms of different stores or planograms of one store in different periods. Flexible analytical tools will allow you to identify products with excess shelf space and reallocate space towards products with high turnover or profit.
All reports can be exported in Excel format.
GOLD Space Automation– a tool for automatically creating planograms. This solution will be necessary for companies that work with a large number of planograms. It will significantly reduce the time for creating and maintaining planograms and will enable the company to support individual store planograms with a limited number of merchandising department specialists.
By using GOLD Space Automation you will be able to create individual planograms for each store using a single set of rules, optimize sections whose design has changed, add and remove products.
GOLD Space Automation– a tool with which you can quickly improve your store display and reduce lost sales thanks to demand-driven placement. The program will offer you a solution that matches your merchandising strategy. This software product from Symphony GOLD will optimize displays specifically for you based on business indicators of goods in stores, reduce response time to strategic and tactical decisions, and reduce inventories and losses. A friendly interface will help the user quickly understand the program.
Optimization of shelf space is aimed at increasing sales, optimizing costs for storing goods, and freeing up frozen funds. Initially, customer demand was met by expanding the retail space and product range. However, like everything in business, shelf space and the amount of money invested in inventory are limited resources.
The shelf space management system allows you to intelligently optimize and automate the management of shelf space and sales floor, and monitor KPIs.
Monitoring (profit per square meter of shelf, etc.) and optimization of shelf space allow sellers and suppliers to increase sales and profits, satisfy customer needs without physically expanding the shelf. A store shelf has limited space, so it is important to present an assortment that will increase turnover and maximize revenue from the store shelf. The most effective so-called “golden shelf” is the space at the level of the buyer’s eyes and hands (at a height of 1.2-1.7 m from the floor), as well as to the right of the planned flow of customers.
To optimize the distribution of shelf space, it is recommended to study the demand of customers and partners, and use modern retail equipment.
Systems for managing store shelf space, which include ABM Shelf, help place only those product categories that are in demand, increase sales, profits, assortment profitability, and optimize the distribution of shelf space. The implementation of shelf space management systems also allows you to:
- reduce the need for discounts, reduce the inventory of goods and the costs of transporting goods,
- simplify managerial decision making,
- optimize the distribution of shelf space,
- regulate relations with suppliers.
Let's define the concepts
SKU(Stock Keeping Unit, literal translation from English - stock retention unit) is one unit of a group of goods or brands, presented in one type of packaging and container (for example, one brand of kefir may contain several units of different degrees of fat content 0.5%, 1% , 2.5%).
Facing- a product that is visible and within the access area for buyers.
Assortment goal— determine the number of product positions that the manufacturer or supplier would like to present on the shelf of the outlet.
The entire assortment, according to customer loyalty, is divided into: 20% priority items, which are sold 3-5 times more often, regardless of price, 60% main stable items in constant demand, and 20% additional items.
Optimize the turnover rate, ensure a high probability of purchase, increase the visual perception of the product - shelf space goals.
The foreign merchandising rule “space to sale” states that the facing of a trademark should occupy the same percentage of shelf space that it occupies in sales of all goods displayed on the retail space. Compliance with this rule contributes to the uniform removal of goods from the shelf, reducing labor costs to maintain product display.
Video review of the retail chain FIRKAN, which implemented ABM Shelf to optimize shelf space
How to determine the optimal display size and how the ABM Shelf shelf space management system can help
The sum of the areas of all planes intended for displaying goods on the sales floor of a store allows us to obtain the total display area.
Space for goods on the shelf, identifying missing goods, determining delivery days, taking into account customer requirements, overall dimensions, seasonal fluctuations and moments of increased demand, can be measured in linear meters, square meters and cubic meters.
- Place high-demand products at customer eye level.
- The higher the weight of the product packaging, the lower on the shelf it should be placed.
- Place new products slightly above customer eye level.
- Place fashion and expensive goods on the top shelves.
- Place products with expiration dates closer to the buyer, and those with later expiration dates - deeper on the shelf.
- Price tags should be easy to read and include accurate information about the price and product.
- Provide easy access to goods.
- The vertical arrangement of homogeneous goods improves visibility.
- The display should be diverse in assortment, colors, and sizes.
- Make changes to product locations less often.

The figure on the right shows vertical placement of homogeneous products, which is more effective for quick orientation and ease of selection of goods.
Functions performed by the system for optimizing shelf space:
- Visual design of planograms of the sales area and shelves, with various configurations and complexity
- Planogram history archive
- Approval of planograms
- Centralized and decentralized management of product display
- Using the characteristics of commercial equipment
- Application of different algorithms to calculate the display of goods: horizontal and vertical arrangement of goods, package dimensions, trademarks, results of ABC analysis of assortment
- Visualization of sales results on planograms of halls and shelves
- Powerful analytical unit for distributing shelf space among racks, product groups, and stores
- Exchange with AutoCAD
Is this functionality for optimizing shelf space often present in the store management accounting system? however, using a specialized service allows you to use many more functions and management options.


The second option is to calculate the number of SKUs while simultaneously adjusting (if necessary) the amount of shelf space allocated for a product category or subcategory. Of course, this method is more labor-intensive, but also more accurate. In this case, it is first necessary to analyze the efficiency of retail space, comparing the share of the category or subcategory in income with the share in the sales area (for large formats) or with the share in the installed area of the store (for small formats). Normally, there should be an approximate correspondence of these shares (for more details, see Chapter 3). Next, having calculated the optimal amount of shelf space, we determine the optimal number of SKUs. Third, calculating the economically feasible number of SKUs. To do this, you need the same data that is used for ABC analysis - shares with a cumulative total by SKU. Let's look at an example from real practice (Fig. 2. 6).
Optimizing shelf space
A sales representative radically resolved the issue of shelf space for his assortment in one of the retail outlets, moving the competitor’s assortment to a half-empty bottom shelf, practically invisible to customers. In response, a sales representative of a competing company began throwing the offender’s assortment off the shelves in all retail outlets in the territory.
A war began, during which both sales representatives were more occupied with restoring their displays than selling new assortment. A meeting in one of the stores led the warring parties to mutual assault, after which they managed not only to come to an agreement, but also to form partnerships to protect their shelf space from the actions of sales representatives of third companies... A concept close to Shelf Share is Forward Stock Share, i.e. .
Shelf share
The entire assortment, according to customer loyalty, is divided into: 20% priority items, which are sold 3-5 times more often, regardless of price, 60% main stable items in constant demand, and 20% additional items. Optimizing the turnover rate, ensuring a high probability of purchase, increasing the visual perception of the product are the goals of shelf space.
Important
The foreign merchandising rule “space to sale” states that the facing of a trademark should occupy the same percentage of shelf space that it occupies in sales of all goods displayed on the retail space. Compliance with this rule contributes to the uniform removal of goods from the shelf, reducing labor costs to maintain product display.
Encyclopedia of Marketing
Attention
If a person has been given a prescription for a certain drug, then, as a rule, he simply approaches the pharmacy employee and asks whether the drug is available. If such a drug is not on sale, it means that the person visits another pharmacy or decides to replace the drug prescribed in the hospital with another drug with the help of a pharmacist.
In this case, it would be quite logical to remove from the counter space those SKUs whose impulse purchase has a minimal probability. As a result, space in the display space will be distributed among those groups and positions of goods that the consumer can decide to purchase independently, without the help of a pharmacist.
Product groups such as fabrics, shoes, clothing, stationery, dishes, wallpaper, tiles, and so on also contain a huge number of SKUs.
Assortment and organization of store shelf space
The saga of six acres (from the book “Modern Supermarket”) One person acquired six acres of land and thought that there was no need to build a classic house-sauna-gazebo on this land, but to engage in commercial activities. He went to the market, talked with the sellers and decided to grow and sell apples.
Actually, as I decided, I did just that, bought and planted an orchard with apple trees on my own six acres of land. The harvest turned out to be quite good, and in the fall the man received good money for it. However, when he was selling his apple crop at the market, he noticed that pears were also a fairly popular product among consumers. The man spent some of the proceeds on purchasing pear trees, after which he began planting them near apple trees.
Entrance
The average width of one juice package is 8 cm, that is, 12 packages can be placed on one shelf, and 60 packages on a rack. Of course, we cannot place 60 SKUs on 60 “seating” places, since some of the most popular positions must receive doubling or tripling of facings (one facing is a line of goods of the same name (SKU) one package wide, placed from the edge of the shelf, facing to the buyer, in the depth of the shelf in the amount of several pieces). As a rule, stores set the average number of facings per SKU at 2 or 3 or more (for a discounter); supermarkets can make a calculation based on 1–1.5 facings per SKU. Let's consider the procedure for calculating the number of SKUs based on the capacity of commercial equipment of an existing store. It should be noted here that there are two options.
Shelf space management
However, for such an adjustment it will be necessary to “pay” in a certain sense. The seller or merchandiser will need to replenish the stock of high-priority items more often.
Info
In the long term, such an approach can be considered completely justified in those stores that rely on the breadth of their product range. In other retail outlets, the decision to reduce the number of SKUs in order to increase the facing of high-priority and main positions has a fairly simple explanation.
Of two evils, you have to choose the lesser - let the store lose two customers who prefer plum or pomegranate juice rather than seven customers who prefer orange drink. Merchandising training It should be said that analyzing sales and making decisions about the need to distribute shelf space is a rather complex process.
Further, within each product group, the shares of categories are determined, for example, gold products without inserts are divided into: chains - 25%; pendants – 20%; rings – 20%; earrings – 15%; bracelets – 10%; necklace – 7%; piercing - 3% The next important point is the identification of the main characteristics of the product, the same ones that are the selection factors in the tree of consumer decisions. The classifier, limited to 3-5 levels of hierarchy, does not cover all the important properties of the product - the characteristics of the product should be reflected in the product card in the information company system. This is necessary to analyze sales in terms of all important characteristics and, accordingly, adjust and form the assortment of goods within a category and subcategory. Let's look at the characteristics using the example of the subcategory “Washing powders” in the category “Laundry Detergents” (Table 2. 5) Table 2.5. Non-food products.
Calculation of the cost of shelf space
In the second case, potential SALES are measured: after all, the buyer purchases a unit of product (facing, and not a certain number of centimeters). Therefore, if the goal of visual dominance is achieved, it is necessary to move on to the goal of dominance in sales and evaluate in faces, and not in centimeters. The relationship between facing and SKU (goals for assortment and shelf space) Facing plays the role of some conventional unit of measurement for shelf space. In this regard, when setting goals for the assortment, it is necessary to understand the CAPACITY of the shelf space, measured in facings. If the assortment goal (number of SKUs) exceeds the shelf space goal (number of faces), then the store will not be able to display the entire assortment at the point of sale. This will entail lost profits and freezing of money in products that are not sold.
Since the merchandising system is one of the main components of sales promotion and, as a result, the successful operation of the store, it is important to constantly evaluate its effectiveness. Let's consider the main indicators of the efficiency of using retail space: o turnover (revenue) per square meter of retail space; o profit per square meter of retail space.
It is these two indicators that should be monitored: turnover and profit. Often, store management only analyzes turnover per m2.
This is acceptable if you need to compare two stores with the same assortment and approximately equal area. But for a detailed and complete analysis, information is needed on the efficiency of using retail space for each product category, and in this case both indicators should be used.
Current page: 9 (book has 49 pages total) [available reading passage: 12 pages]
Font:
100% +
System tools for optimizing retail and shelf space
Sometimes you can come across the statement that the literature on the efficiency of organizing retail and shelf space is divided into three categories. This opinion, in particular, is shared by the outstanding researcher in this field, Marcel Corstjens. These three categories are: reports of empirical studies (like those we have discussed in this chapter); books on the commercialization of research developments, namely: on electronic merchandising software products; and academic work by mathematicians and statisticians seeking to optimize models for organizing shelf and retail space.
Planogram software products often rely on the rule of thumb that product placement is determined based on the profit or sales volume it generates. Similar solutions have been offered on the market since the 1970s; Among the first were the SLIM (Store Labor and Inventory Management) and COSMOS (Computer Optimization and Stimulating Model for Supermarkets) systems. I did not set out to give a complete overview of all the algorithms developed since then, up to modern tools for constructing planograms, but I considered it necessary to describe the most important milestones in their evolution. It should be noted that many of the commercial tools are created by researchers who prefer the accumulation of capital to the accumulation of knowledge, so their developments are often simplified versions of the optimization models found in academic papers.
I will focus here on the latter as they always precede commercial decisions. To be useful in practice, programs like Spaceman and Appollo must be based on a significant simplification of reality—a detail that seems to be of little concern to the research community.
Three key milestones, which will be briefly described below, show how researchers gradually solved the optimization problem by incorporating the following new factors:
Different elasticity of display of different product lines;
Cross elasticity of the layout;
Direct commodity costs.
Different product lines have different display elasticityEvan Anderson and Henry Amato (1973) developed one of the first algorithms to solve the shelf space optimization problem. As they say among marketers, they approached the problem “from the demand side.” The researchers proceeded from the knowledge that was available at that time, namely from the fact that different product lines have different elasticity of display. Simply put, their model was based on logistic regressions that calculated beta coefficients for different product lines. It is this type of computing that underlies the aforementioned SLIM and COSMOS systems.
Cross display elasticity and direct commodity costsThe next important step was taken by the Frenchman Marcel Corstiens and the Englishman Peter Doyle (1981). The same Peter Doyle, who, if you remember, criticized research in the field of retail marketing for its lack of progress. The model they proposed was more extensive than previous ones and is still discussed to this day. Among other things, they included the ability to calculate direct commodity costs (related to the acquisition, storage, and lack of goods on shelves, the so-called out-of-stocks), demand effects and cross-elasticity factors. It was the inclusion of the latter indicator that brought fame to their model.
They tested their model across five product lines in 140 stores selling candy, ice cream and gift cards, with $30 million in annual sales. The elasticity of the layout was on the order of 0.19, the researchers reported, and thus consistent with what earlier experiments had shown. It was also found that the cross display elasticity was negative between different types of sweets (if stores sold more chocolate, the demand for caramel fell) and positive between sweets and gift cards.
In addition, direct merchandise costs associated with purchasing (ordering and transportation), handling (storage, insurance, and product losses), and stock-out-of-stocks were calculated. The calculations were based on average data from 10 stores, but were used for all outlets covered in the study. According to the findings, higher turnover items (such as chocolates versus gift cards) incur higher processing costs.
Next, M. Corstjens and P. Doyle performed calculations for planograms (1) currently used in stores, (2) developed based on sales data, and (3) developed based on gross profit; to compare them with the results of your new model. The comparison showed that the latter potentially provides $128,000 more in net profit than currently used planograms, $104,000 more than planograms based only on sales data, and $97,000 more than planograms based on gross profit. This was primarily because rule-of-thumb models allocated too little space for additional products such as ice cream and gift cards. In percentage terms, this meant an increase in net profit of less than 0.5%.
Lack of goods on shelves ( out-of-stock) is a serious problem for retailers. Customers react to this in one of five ways: 1) go to another store, 2) delay the purchase, 3) abandon the purchase, 4) buy a different size package or a similar product of the same brand, or 5) switch to another brand. David Grant and John Fernie (2008) report that a 2003 study by IGD found that 65% of UK shoppers choose one of the first three options when a product they want is out of stock.
Cannibalization effectThe idea of this type of cross-elasticity was proposed by the French researcher Alain Boultes, and his attempt to incorporate the cannibalization effect into planogram models was successful. In other words, he was the first to develop a good solution for calculating the decline in sales of Brand B as a result of Brand A gaining more shelf space and showing increased sales. A. Bultes' model is called SH.A.R.P. and still remains functional (see below), as shown by testing in Belgian grocery stores.
At first glance, including the cross-elasticity factor in the model seems trivial, but everything is not as simple as it seems. How complementary and/or competing are, for example, rice and spaghetti? Add millet, potatoes, french fries, other grains and root vegetables to this equation, and the complexity increases exponentially. Keep in mind, however, that cross elasticity varies across product pairs, as well as across time and situation. Products that compete with each other in one situation (burgers and meatballs might be considered alternatives for dinner) turn out to be complementary in another (if you are inviting friends over for a barbecue).
Competition with rules of thumbOnce the fundamental models were developed, researchers devoted their energies to further optimizing them, often by eliminating various limiting conditions. For example, if an earlier model included a cross elasticity factor ( M. Corstjens and P. Doyle 1981, 1983), then the later excluded it from consideration in order to focus on another aspect, such as, for example, vertical or horizontal placement ( A. Lim, B. Rodriguez and K. Zang, 2004). Much time has been spent trying to mathematically solve the problem of why the packaging of different products looks the way it does. Of course, mathematicians are not used to taking into account certain facts, for example, that some products (the same pack of coffee) by definition must have a larger package than others (a packet of yeast).
On the one hand, the models suffered from not being simple enough to be used in practice. On the other hand, interest in them never waned. Researchers have continued to try to create efficient algorithms for optimizing shelf space that can compete with rules of thumb that allocate facings based on share of total sales or gross profit. In 1988, Frenchman Alain Boultes and Belgian Philippe Naert introduced a model called SH.A.R.P. (Shelf Allocation for Retailers’ Profit - distribution of shelf space for retailers’ profits). They argued that it far exceeds the empirical principle " display area / share of sales". However, a year later A. Bultes had to swallow a bitter pill. It turned out that after including the cannibalization effect (SH.A.R.P. II) in the model, most of its advantages over this rule of thumb disappeared ( A. Bultes et al., 1989). However, A. Bultes estimates that a store will lose approximately 2.7% of gross profit if it does not optimize shelf space using SH.A.R.P. II. As a result, due to their ability to provide a level of profitability comparable to the entire net profit received by the retailer, optimization models continue to attract keen interest from researchers.
Return on efforts to ensure efficient use of retail spaceSo far, I have introduced you to scientists who were pioneers in introducing a new approach to developing planograms. Among their modern followers, I would like to name the talented researcher Ming-Hsien Yang from Taiwan. He has developed models to reduce the computational power required for optimization algorithms and conducted cost-effectiveness studies on planograms.
Together with his colleague ( M.-H. Yang and W.-C. Chen, 1999) he conducted a study whose purpose was to study how retailers work to effectively use retail space. Store owners were asked to answer questions about how much time and effort they spend on (1) strategic and (2) operational work. Each item (strategic/operational work) contained five questions. The researchers then correlated the responses with the retailers' economic indicators: total sales, sales per square meter, profit per square meter.
A clear pattern was found: the quality of operational work to ensure the efficient use of retail space was reflected to the greatest extent in sales per square meter, while the quality of strategic work influenced the amount of gross profit per square meter of retail space.
Table 3.13. The table shows the results of an ANOVA study using f-values (and p-values). It appears that retailers' efforts at both the strategic and operational levels to improve the efficiency of their retail space are paying off.
In a recent study, Chase Murray, Abhijit Talukdar, and Debu Gosavi (2010) developed an optimization model that takes into account factors such as product prices, shelf placement, number of facings (display area), and packaging orientation. C. Murray and his colleagues report that their shelf space management techniques resulted in sales improvements in the same ranges as in the previous studies we reviewed. However, they argue that many of the models used today represent a significant abstraction compared to the real context in which a retailer makes decisions. The development of 3D modeling to create planograms is certainly an important step forward, especially for those product categories where the packaging does not have a natural front face.
Despite the efforts of researchers such as C. Murray and colleagues (2009) to create more realistic models, many retailers and manufacturers use planograms merely as preliminary sketches of how shelf space might be organized, but never fully rely on them. Store owners modify them by including factors such as storage specifics, type of outlet, communication goals (for example, better space and more space may be allocated to those goods that retailers want to sell, rather than those that are already in demand), adjacent location of related product categories, etc. All this ultimately has a great impact on the final appearance of planograms. As well as the empirical principle of allocating shelf space depending on the product’s share of sales (or gross profit).
ConclusionReview of the study by M.-H. Yang and W.-C. Chenya, I conclude this section on optimization models. Based on all of the above, we can draw the following conclusion: efforts to optimize retail space certainly pay off in the form of increased profits and sales, but there is a certain limit to how much time and effort a retailer can spend on this activity. Therefore, despite the advent of increasingly advanced commercial software tools for retail space planning, challenges remain. In particular, they are related to the estimated values that the model requires as input. For example, these estimates relate to cross-elasticity or any strategic decisions where the marketer must enter subjective data. An interesting study was conducted by Norm Borin and Paul Farris in 1995. Scientists wanted to test how much incorrect numbers could be entered into the model without noticeably affecting the result. Having tested SH.A.R.P. II, they found that input values that depend on the subjective judgment of decision makers can deviate significantly from the actual values (up to 50%) without the model yielding to rule-of-thumb techniques.
Another, perhaps more important problem is that optimization models do not take strategic decisions into account. The algorithms are based on historical data, but a retailer may want to influence the behavior of its customers by reorienting them from the products they buy today to some others. We will discuss this problem in the next section.
Strategic decisions regarding store private labelsResearch in the field of shelf space optimization is becoming increasingly applied. As an example, two of them relate to private label networks and their placement on shelf space. The first study was conducted by Marcel Corstjens and Rajeev Lal (1994). They described the difference in the approach of European and American grocery stores to dealing with private labels. The first ones are allocated under private label the most profitable locations, often exceeding the share of these products in the local market, while the latter mainly concentrate their brands in low-price segments.
In their study, M. Corstjens and his colleagues clearly showed that in most markets it is preferable to work according to the European model. This places certain demands on the quality of products private label and the pricing policies of their national competitors, but in light of our discussion, the most important point is that the strategic solution proposed by M. Corstjens cannot be implemented using existing planogram software tools. Meanwhile, the researchers insist that such a solution should entail all the necessary steps for its implementation, right down to the appropriate organization of shelf space.
I prefer to maintain my role as an objective observer and not express my opinion on this issue here. Thus, in another applied study, Fernandez Nogales and Gómez Suarez (2005) compared how much shelf space different stores allocated to their brands (the study covered the periods from 1998 to 1999 and 2003). The results obtained confirmed the conclusion of M. Corstjens and his colleagues that private label get more shelf space than they “deserve” based on their market share. Interestingly, the researchers also looked at overall product lines where stores heavily promoted their own brands and found that this impacted their profitability, although not all stores experienced the same negative impact. As a result, some retailers have begun to reduce the display space of their own brands in order to maintain sales. On the other hand, they still continued to allocate a lot of space for new private label.
The conclusion is this: it is not at all difficult to influence the decisions of buyers by appropriately organizing shelf space; the main thing is not to abuse this tool, even though buyers are rarely aware of the changes taking place.
Comparison of display elasticity between different store departments
Of course, this issue is closely related to the topic of sales floor layout, which will be discussed in chapter 8, but I decided to discuss it here because it directly relates to the elasticity of the display.
Two French researchers, Pierre Desmés and Valerie Renaudin, followed R. Kerhan (1972) and conducted a large-scale study to try to establish the causes of the elasticity of the display. But, unlike R. Kerhan, the French decided to compare not product lines, but different departments within one outlet. In 1998, they published a paper on the relationship between dedicated retail space and sales across different store formats and departments. The study covered about 200 universal shops in France.
Scientists have hypothesized that the elasticity of the display is influenced by the type of outlet, as well as the product. They examined the differences between three different store formats in a selected chain (small, medium and large) and also categorized the entire range of products by department (from jewellery, fashion and home goods to six types of grocery departments).
The study showed a pretty clear picture. As it turns out, there are significant differences in the elasticity of the display between departments. The highest values of this indicator are typical for such goods as underwear, jewelry, fruits and vegetables. Therefore, allocating more space to these departments is most cost-effective. Negative elasticity was found for fashion products, and most of the assortment was found to be relatively inelastic. In the theoretical part of the article, reference was made to a thesis of a German researcher, in which, based on the results of more than twenty experiments, it was concluded that approximately 40% of the assortment in German supermarkets has a display elasticity of less than 5% (if you remember, the rule of thumb says about 20%).
Lessons for retailersRetailers can also learn a number of important lessons from this body of research. As with private labels, success depends on making the right strategic decisions. For example, a study by P. Desme and V. Renaudin showed that fashion products have a positive display elasticity in larger stores. In all likelihood, the latter can create an atmosphere that encourages people to purchase clothes. At the same time, in smaller retail outlets the same product category shows negative elasticity. Thus, it is important for a retailer to know exactly what to expect from a particular store format. If the researchers had not separated outlets by type, the above differences might not have been as obvious and might have gone undetected. One lesson for retailers is that current shelf space optimization tools may work well for some store formats and product categories, but not others.
Kerhan (1972) outlined another reason to be careful when calculating the elasticity of a layout. The fact is that in many cases this indicator increases as the display area decreases and decreases as it increases. A similar trend is found in the case of price elasticity, where empirical studies indicate the nonlinear nature of the demand function. This means that an increase in sales due to a price decrease is not the same as a decrease in sales due to a price increase. In our case, this means that it is often impossible to reduce the area of any department, even if it has low elasticity of the layout.
The training program included the latest CM methods and tools - planning and control methods; analysis and optimization of the pharmacy chain’s assortment; managing the profitability of retail and exhibition space; developing and implementing a category development strategy and much more. So, let's take a closer look at the main QM analyses.
ROLE ANALYSIS
This analysis is used to assess the sustainability and competitiveness of the category. It is carried out by categories and groups of homogeneous goods (types, brands). The dynamics of role analysis reflect the rotation of assortment in the category. The category contains all products of 6 main roles (Fig. 1). After conducting a self-conducted role analysis for specific product categories, participants found that in most cases the categories did not contain any of the 6 main role products (either protector or cash generator). In order to correctly conduct a role analysis, it is necessary to determine the trade margin and turnover for each brand and assign a role (Table 1). Next, based on the obtained values, you should build a graph (Fig. 2) and analyze whether the markup and role are correctly determined for all positions.
Brand | Trade margin, % | Role meaning | |
---|---|---|---|
Brand A | 8 | 1 860 | Flow generator |
Brand B | 25 | 1 500 | Cash generator |
Brand C | 25 | 1 040 | Cash generator |
Brand D | 18 | 835 | |
Brand E | 42 | 420 | Profit generator |
Brand F | 36 | 540 | Purchase generator |
Brand G | 15 | 320 | Delta* |
Brand H | 21 | 700 | |
Brand I | 24 | 800 | |
Brand J | 45 | 120 | Image generator |
Total | 8 135 |
Delta is a brand or product position located in the lower left corner of the matrix. Delta meets the standards of the EPP retail network
At the first stage of the analysis, the “sustainability” and “sufficiency” of the assortment are assessed.
It is known that in order to generate maximum turnover and profit, the category requires the presence of goods of 6 main roles.
Flow generators are branded, branded products that are well known in the market due to active marketing by the manufacturer.
Since these goods are presented in all retail chains in the city, the consumer, as a rule, has a good understanding of sales prices. Accordingly, the markup for these goods is set at the level of “average” or “slightly below the average” for the market. The main task of flow generators is to generate a flow of customers to stores. Trade turnover for flow generators is high and above average in the category.
Cash generators are lesser-known, perhaps unbranded, products that are analogous to flow generators.
Retail prices for the cash generator are slightly lower than for the flow generator; but the markup is much higher. The manager's task is to raise sales of the cash generator to the level of the flow generator and higher.
Purchase generators - the entire range of goods of impulse and passive demand; related products.
Purchase generators have an “above average” markup for the product category and work to increase overall profitability and increase the number of purchases in a receipt.
Profit generators are products with high markups and a significant share of sales in the product category. Most generators in the category work to support them.
Image generator(s) - products that allow you to position the category range on the market and in the minds of consumers. May have the following characteristics:
- maximum markup, insignificant sales volume (rare, exclusive goods); available in the supermarket assortment;
- minimum markup, significant sales volume (consumer goods, “loss leaders”); available in the discounter's assortment.
Defender is a product sold only by one retail operator in the region (exclusive contract, private label of the chain) or on unique terms. Allows you to retain the main pool of consumers even with the aggressive assortment policy of competitors. It may have fundamentally different characteristics in terms of markup and sales volume.
At the second stage of the analysis, the area of the figure is estimated using role analysis. An increase in area may indicate:
- changes in sales volume of generators in the category;
- changing the trade markup for category generators;
- the emergence of new generators, that is, the rotation of the assortment.
Most changes in the area of a figure according to role analysis are associated with the emergence of new (additional) generators in the category. The manager either immediately introduces the leading product with the maximum turnover and (or) markup into the category; or introduces a new product into the range, planning that someday it will move to the upper right part of the matrix.
But since store shelves are “not rubber,” introducing new products into the assortment is only possible if inappropriate product items are removed from the assortment matrix (lower left corner according to role analysis).
Thus, an increase in the figure area guarantees constant rotation of the assortment and confirms that the increase in turnover and profits occurred not only due to marketing, consumer market growth or inflation, but also due to optimization of the assortment structure.
structural analysis of the assortment. optimal depth
How to calculate the number of required product items (SKU-Stock Keeping Unit) included in the category at which the pharmacy will receive maximum turnover or maximum turnover? In addition to ABC analysis, this can be done using structural analysis, which the training participants also performed in practice. Structural analysis allows you to calculate the optimal structure of the assortment, that is, the number of groups, subgroups, brands, articles; their renewal and ratio, at which maximum turnover and profit are achieved.
Structural analysis is based on the calculation of the following assortment indicators:
- category breadth - the number of groups included in the category (subgroups, species, varieties);
- category completeness - the number of brands included in the category (group, subgroup);
- category depth - the total number of product items (articles, SKU);
- category harmony - the degree of similarity of product items in the price range;
- category stability - fluctuations in the main indicators of the category assortment for the analyzed period;
- category renewal - the number of new product items in the assortment structure (for the analyzed period).
At the first stage of the analysis, the optimal values of the indicators are calculated. To do this, the weekly values of turnover (or any other indicator, for example, profit, turnover) are compared with the corresponding value of the assortment indicator. Depth data (number of SKUs) provides information about the products that were in stock in a given week. The comparison is made at least 12 weeks in advance (Table 2). Based on the results of data comparison, a dot plot is constructed (Fig. 3). A trend line (polynomial trend) is added to the graph. The transition point is determined on the trend (after which the growth in trade turnover slows down). The transition point is the optimal value of the indicator for a given season.
A week | Depth, number of sku | Weekly turnover, thousand rubles. |
---|---|---|
1st | 102 | 5300 |
2nd | 80 | 3500 |
3rd | 110 | 4800 |
4th | 90 | 4500 |
5th | 75 | 3750 |
6th | 85 | 4250 |
7th | 100 | 5400 |
8th | 142 | 5100 |
9th | 125 | 4700 |
10th | 80 | 4000 |
11th | 135 | 5000 |
12th | 85 | 4250 |
13th | 135 | 4400 |
14th | 110 | 4870 |
15th | 130 | 4800 |
16th | 140 | 5050 |
16th | 118 | 4100 |
In this case, the optimal category depth is in the range from 110 to 120 SKU (for a particular season).
Structural analysis should be calculated once a season. After calculating the optimal assortment indicators, the category manager reports weekly to the commercial director for deviations of the current indicators from the optimal ones. It is recommended to use the analysis for a homogeneous group of products.
harmony in price level
How to determine how many SKUs should be in the low, medium and high price segments in a product category? The traditional method of determination is by demand. And under what structure will we get maximum turnover or income for a specific product category? Analysis of the harmony of the assortment by price level (analysis of the price line) by category and subgroup allows us to determine whether the cost of brands in the category is harmonious.
The result of the calculation is the value of harmony for 1 week for specific products. As an example, the beer product line was chosen (Table 3). At the first stage, it is necessary to list all product items included in a specific product group. Next, you should choose a product with the minimum price - in this case, it is the Stary Melnik beer with a cost of 18 rubles. And then calculate the ratio of the cost of each product and the minimum price and determine the arithmetic average. Thus, to analyze harmony by price, you need to know:
No. | Name, “beer, glass, 0.5” | Cost, rub. |
---|---|---|
1 | Baltika No. 3 | 20 |
2 | Baltika No. 5 | 25 |
3 | Baltika No. 5 | 28 |
4 | Old Miller | 30 |
5 | Klinskoe light | 24 |
6 | Klinskoe dark | 27 |
7 | Staropramen | 32 |
8 | Zhigulevskoe | 34 |
9 | Old Miller | 18 |
10 | Heineken | 38 |
- optimal value of the characteristic (sign);
- the ratio of each characteristic to the optimal characteristic;
- the arithmetic mean over all ratios (over all division results).
Thus, a formula for price harmony was derived using the example of beer products: Gm (Ai) = (20 / 18 + 25 / 18 + 28 / 18 + 30 / 18 + 24 / 18 + 27 / 18 + 32 / 18 + 34 / 18 + 18 / 18 + 38 / 18)/10.
In this case, the price harmony was 1.5. If harmony is about 1, this means that all goods are sold at the same price and the needs of one price segment of consumers are satisfied. The greater the harmony - 2, 10, 15 or higher, the greater the difference in prices, which means it is possible to satisfy consumers in different price segments. Optimal harmony is the one at which you can get maximum turnover or profit. One indicator of harmony for 1 week (1.5) is not enough. In the same way, it is necessary to calculate the harmony for the 2nd, 3rd, 4th week, etc. Based on the results obtained, a structural analysis should be carried out to see on the graph the point at which maximum turnover or income can be obtained for a given variety.
The number of weeks should be about 16 (as many weeks as there are in the season), then you need to calculate the arithmetic average. For example, if the optimal value - the peak point on the graph - is 5, and in the current period the harmony is 4.5, then the category manager manually, according to role analysis, includes or displays some product items in order to “pull up” the harmony indicator to 5. Harmony is necessary so that in the future, when introducing or introducing any product categories, the products will not be positioned.
ABC analysis
“If you want to kill the assortment, do ABC analysis,” V. Snegireva told her students. But this statement did not shock them. ABC analysis is a statistical method of analysis based on Pareto's law - a smaller number of causes are responsible for most of the results. ABC analysis allows you to understand which products are sales leaders and which, on the contrary, are outsiders. But ABC analysis will never show why some product items moved up in the rankings, while others moved down. Perhaps the category managers did this on their own, moving their positioning areas, or perhaps it was caused by a seasonal decline (or if it was a new product, etc.). And most importantly, the goal of ABC analysis is to optimize the product range. It is logical that those positions that are at the bottom of the list in terms of share in the category should be excluded. Such eliminations can be carried out weekly, but as a result they can be further optimized until only one product item remains. V. Snegireva showed photographs taken in a supermarket, where the assortment of the “sunflower oil” category consisted exclusively of “Oleina” oil. ABC analysis is necessary only for determining and controlling inventory, but not for managing sales. ABC analysis should only be used in conjunction with XYZ analysis.
ABC analysis with merchandising index
How to understand what influenced the increase in sales of over-the-counter products or their decrease? This requires ABC analysis with the merchandising index - ABCi, which allows you to take into account factors such as the number of the shelf on which the brand is located and the share of shelf space occupied by the brand in the group. It allows you to see how brands could sell if they were displayed at the same height above the floor and occupied an equal position on the shelf.
The merchandising index helps to equalize sales from different shelves. It shows how items would sell if they were on the same shelf. As a rule, the bottom shelf is equal to 1.4, the next one up is 1.0, at eye level 0.8 or 0.6, the top - 1.2. If the equipment is three-shelf and 40 items were sold from the 1st shelf, 100 from the 2nd, and 80 from the 3rd. The total is 220 items, which equates to 100%. Next, you should calculate the share of each shelf, which will be the merchandising index. ABC analysis formula with merchandising index:
АВСм=Т · Im · 1 / TO, Where
T- current trade turnover;
Im- merchandising index;
TO- the share of exhibition space occupied by a product (brand) on equipment.
Brand, SKU | Brand turnover, thousand rubles. | Brand share in group turnover | Share from accumulated as a result | ABC analysis group | ABCi analysis group |
---|---|---|---|---|---|
Brand A | 420,00 | 0,14 | 0,14 | A | C |
Brand B | 380,00 | 0,12 | 0,26 | A | B |
Brand C | 340,00 | 0,11 | 0,37 | A | B |
Brand D | 290,00 | 0,09 | 0,47 | A | C |
Brand E | 270,00 | 0,09 | 0,55 | B | C |
Brand F | 250,00 | 0,08 | 0,63 | B | B |
Brand G | 230,00 | 0,07 | 0,71 | B | A |
Brand H | 220,00 | 0,07 | 0,78 | B | C |
Brand I | 180,00 | 0,06 | 0,84 | C | A |
Brand J | 165,00 | 0,05 | 0,89 | C | C |
Brand K | 130,00 | 0,04 | 0,93 | C | B |
Brand L | 120,00 | 0,04 | 0,97 | C | A |
Brand M | 80,00 | 0,03 | 1,00 | C | C |
Total | 3 075,00 | 1,00 |
Brand, SKU | Brand turnover, thousand rubles. | Layout, linear. meters | Shelf number | Merchandising Index, Im | Share of space on the shelf, K | Comparable values | Share in comparable amounts |
Share with cumulative total |
Group, according to the results of ABCi analysis |
---|---|---|---|---|---|---|---|---|---|
Brand L | 120,00 | 0,50 | 1 | 1,4 | 0,02 | 8 400 | 0,162129745 | 0,162129745 | A |
Brand G | 230,00 | 1,00 | 5 | 1,2 | 0,04 | 6 900 | 0,133178005 | 0,29530775 | A |
Brand I | 180,00 | 1,00 | 1 | 1,4 | 0,04 | 6 300 | 0,121597309 | 0,416905059 | A |
Brand F | 250,00 | 1,00 | 2 | 1 | 0,04 | 6 250 | 0,120632251 | 0,53753731 | B |
Brand K | 130,00 | 1,00 | 1 | 1,4 | 0,04 | 4 550 | 0,087820279 | 0,625357588 | B |
Brand B | 380,00 | 2,00 | 4 | 0,8 | 0,08 | 3 800 | 0,073344409 | 0,698701997 | B |
Brand C | 340,00 | 1,50 | 3 | 0,6 | 0,06 | 3 400 | 0,065623944 | 0,764325941 | B |
Brand H | 220,00 | 2,50 | 1 | 1,4 | 0,10 | 3 080 | 0,059447573 | 0,823773515 | C |
Brand A | 420,00 | 3,00 | 4 | 0,8 | 0,12 | 2 800 | 0,054043248 | 0,877816763 | C |
Brand J | 165,00 | 2,00 | 2 | 1 | 0,08 | 2 063 | 0,039808643 | 0,917625406 | C |
Brand E | 270,00 | 4,00 | 5 | 1,2 | 0,16 | 2 025 | 0,039084849 | 0,956710255 | C |
Brand D | 290,00 | 3,50 | 3 | 0,6 | 0,14 | 1 243 | 0,023988585 | 0,98069884 | C |
Brand M | 80,00 | 2,00 | 2 | 1 | 0,08 | 1 000 | 0,01930116 | 1 | C |
Total | 3 075,00 | 25,00 | 1,0 | 51 810 | 1 |
The seminar participants first conducted a classic ABC analysis with a merchandising index (Table 4). After that, fill out the table. 5, where we added turnover, linear meters, and shelf number for the product item. The merchandising index was calculated using the formula given above, taking into account 5 shelf space. The comparable values column was calculated as follows:
trade turnover · merchandising index: percentage of shelf space.
Based on the data obtained, a classic ABC analysis was then carried out, where the share was then calculated in comparable values and with a cumulative total, and based on its results, each brand was assigned a group. Participants then compared the results of the classic ABC analysis with the ABC analysis with the merchandising index. If a product item received a different group according to the results of two analyzes (for example, in one A, and in the other - B), this means that it takes up too much space on the shelf. This discrepancy must be used in negotiations with distributors, reducing the display of goods and changing the number of its shelf.
Brand | Brand turnover, rub. | Brand display, cm | EPP, rub. | <ЕПП | >EPP | Sufficiency, cm | Extra, see | Distribution, cm | Brand display 2, cm | EPP 2, rub. | Trade turnover of brand 2, rub. |
---|---|---|---|---|---|---|---|---|---|---|---|
Brand A | 7 800 | 260 | 30,0 | 26,3 | 0,0 | 139 | 121 | 0 | 139 | 56,3 | 7 800 |
Brand B | 4 600 | 100 | 46,0 | 10,3 | 0,0 | 82 | 18 | 0 | 82 | 56,3 | 4 600 |
Brand C | 12 000 | 160 | 75,0 | 0,0 | 18,7 | 0 | 0 | 37 | 197 | 75,0 | 14 812 |
Brand D | 5 400 | 120 | 45,0 | 11,3 | 0,0 | 96 | 24 | 0 | 96 | 56,3 | 5 400 |
Brand E | 16 000 | 200 | 80,0 | 0,0 | 23,7 | 0 | 0 | 48 | 248 | 80,0 | 19 801 |
Brand F | 6 000 | 200 | 30,0 | 26,3 | 0,0 | 107 | 93 | 0 | 107 | 56,3 | 6 000 |
Brand G | 12 000 | 160 | 75,0 | 0,0 | 18,7 | 0 | 0 | 37 | 197 | 75,0 | 14 812 |
Brand H | 9 600 | 160 | 60,0 | 0,0 | 3,7 | 0 | 0 | 7 | 167 | 60,0 | 10 048 |
Brand I | 6 600 | 100 | 66,0 | 0,0 | 9,7 | 0 | 0 | 19 | 119 | 66,0 | 7 886 |
Brand J | 4 400 | 40 | 110,0 | 0,0 | 53,7 | 0 | 0 | 108 | 148 | 110,0 | 16 231 |
Total | 84 400 | 1 500 | 56,3 | 128 | 257 | 257 | 1 500 | 107 391 |
Matrix analysis
Matrix analysis allows you to make a decision on excluding a position from the assortment, as well as on the redistribution of resources (shelf and retail space) between product items included in the same category. Matrix analysis is carried out both by category and by groups of homogeneous goods (types, brands, etc.) At the first stage of the analysis, all product items are compared according to two characteristics:
- The Y-axis shows the weekly (monthly) growth rate of turnover of a specific SKU;
- The X-axis shows the relative share of turnover (RPT) of the SKU (the ratio of the turnover of the SKU to the turnover of the sales leader in the group).
The ODT share is calculated as follows: the sales volume of the product item under study is divided by the sales volume of the leader in a particular segment.
As a result, each product item (SKU) falls into one of four sectors (Fig. 4):
Sector A - high CCT and growth rates of trade turnover (increase in trade turnover - above 1/4 in the trading network; CCT - above 0.6).
Sector B - large market share and relatively stable growth rates (trade turnover growth - below 1/4 of the retail network; CCT - above 0.6). The cash flow they generate significantly exceeds investment needs. But since most of them have moved down from sector A, they maintain a downward trend in the growth rate of trade turnover. Therefore, the manager’s primary task is to smooth out this trend for as long as possible.
Sector D - outsider goods (increase in trade turnover - below 1/1 in the trading network; CCT - below 0.6). The support of these products is justified to the extent that their existence is consistent with the concept of the trade assortment (specialization of the enterprise, image, desire to give the assortment special properties). However, since these elements are at the final stage of the life cycle, they are the ones that are subject to exclusion in the event of underfunding of any of the sectors of the matrix analysis.
Sector C - high growth rates and insignificant trade turnover (increase in trade turnover - above 1/4 in the retail network; CCT - below 0.6). These products have high financing needs and low income levels (due to an undeveloped sales market). Since not all of the elements presented in field B will be able to move into sector A, the question arises of how justified the financing of a particular element is.
Depending on how the goods behave in the matrix (coordinates along the matrix axes increase or decrease; CCT and trade turnover increase or decrease, etc.) compared to the previous period, all elements are assigned one of the financing lines:
1st line - funding priority. Receiving the 1st line of financing involves an increase in the retail and exhibition space allocated for the product (minimum, with a coefficient of 1.7); increasing the number of faces in a display; duplication of goods at additional points of sale, etc.
2nd line - financing of the product item remains unchanged. The amount of resources that the product received in the previous period, it continues to receive in the planned period.
3rd line - financing of goods is reduced to the initial level (to the level prior to the appearance of the 1st line of financing). The display area and the number of facings are reduced, and the product is removed from additional points of sale.
4th line - the product is being prepared for removal from the matrix (possibly temporary). The manager stops placing orders for this product, the remaining balances are sold online.
5th line - the product should be excluded from the assortment matrix (before the onset of the season), unless it is an image product.
6th line - appears for the product only if the product was not on sale for some reason. The appearance of the 6th line requires further investigation.
The decision to exclude a product item cannot be made when only one “5” appears. The manager makes a decision to increase funding or exclude a product based on the results of 4–6 weeks, analyzing the current trend.
The seminar participants also calculated the approximate turnover of the network for the future period, based on its format and area. Its calculation formula is:
T 2008 = (((Tm2 2007 * Sm2 2007 + Tm2 2007 * Sm2 2008 * (b-n)/12) + Inf. (%)) + RPR (%)) + Int. (%), Where, b- months remaining until the end of the year from the opening of the store; n- months allocated for store promotion; Inf.. - inflation percentage (approximately); RPR- growth of the consumer market; Int. - intensive increase in trade turnover.
Opportunities and prospects
The training participants learned how to conduct various types of comprehensive analysis of the assortment, received recommendations for optimizing the category structure, and studied modern methods of assortment management, methods of its analysis and optimization.
It is impossible to talk about such a voluminous topic as product assortment management in one publication. You can get practical lessons on CM at this kind of training. To be fair, it should be said that today there is very little comprehensive information in the public domain regarding CM in the pharmacy business. This is easily explained by the competition in this segment. However, one thing is clear - independent and painstaking work in studying the intricacies of CM will become an invaluable tool for pharmacy specialists in sales management.
Oksana Sergienko